Pose Estimation on NVIDIA Jetson platforms using OpenPifPaf
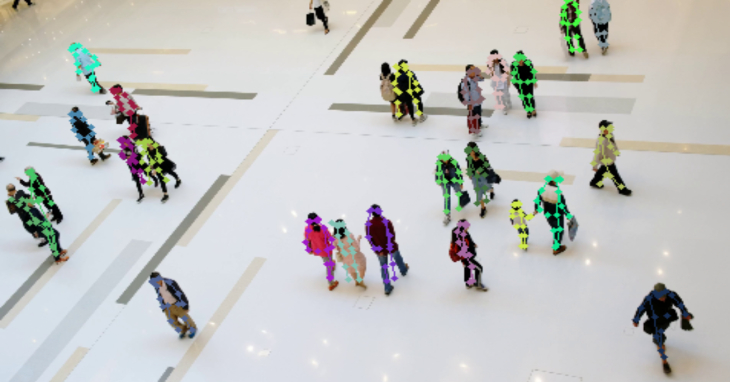
Figure 1. Pose Estimation output on NVIDIA Jetson TX2 using OpenPifPaf.
In this post, we will walk through the steps to run an OpenPifPaf Pose Estimation model on NVIDIA Jetson platforms.
This is the first chapter of our work on Human Pose Estimation. Following are some useful links to explore:
Galliot’s GitHub Repository for this work.
If you don’t know about pose estimation refer to Overview of Human Pose Estimation with Deep Learning.
Introduction to Galliot edge-device-friendly Pose Estimator, TinyPose.
An overview of the Data Labeling Methods, Challenges, and Solutions.
Pose Estimation is a computer vision technique that detects body posture, i.e., the human body’s spatial configuration, in videos or images. Pose estimation algorithms estimate body pose using a set of KeyPoints that indicate key body joints, such as elbows, knees, and ankles.
In this post, we will walk through the steps to run pose estimation on NVIDIA Jetson platforms. Jetson devices are small, low-power AI accelerators that can run machine learning algorithms in real-time. However, deploying complex deep-learning models on such devices with limited memory is challenging. In this case, we need to use inference optimization tools, such as TensorRT, to be able to run deep learning models on these platforms on edge devices.
In this work, we generated a TensorRT inference engine from a PyTorch pose estimation model to run pose estimation on Jetson platforms in real-time. Our model can work well on real-world CCTV data compared to the existing models.
The source code, models, and Dockerfiles introduced in this blog are available for download.
1. Pose Estimation on Jetson Devices; where to start?
To run pose estimation, we searched for and deployed different pre-trained pose estimation models on Jetson devices. Several open-source models were available for pose estimation to experiment with. Let us explain more about a few of them:
1.1. TensorRT Pose Estimation
TRTPose is an open-source project for real-time pose estimation accelerated with TensorRT. Two pose estimator models pre-trained on the COCO dataset are available for download on this repo.
Since the models are accelerated with TensorRT, it was straightforward to deploy them on Jetson devices. We tested both pre-trained models on different sets of data on Jetson Nano, and the densenet121_baseline_att_256x256_B model achieved the best performance with a frame rate of 9 FPS.
As you can see in the example images below, the model worked well on images where people were standing close to the camera (Figure 2). However, it failed to generalize well to real-world CCTV camera images, where people occupy only a small portion of the image and partially occlude each other (Figure 3).
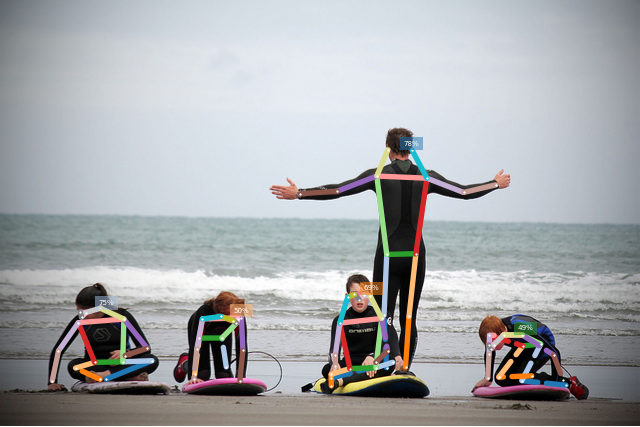
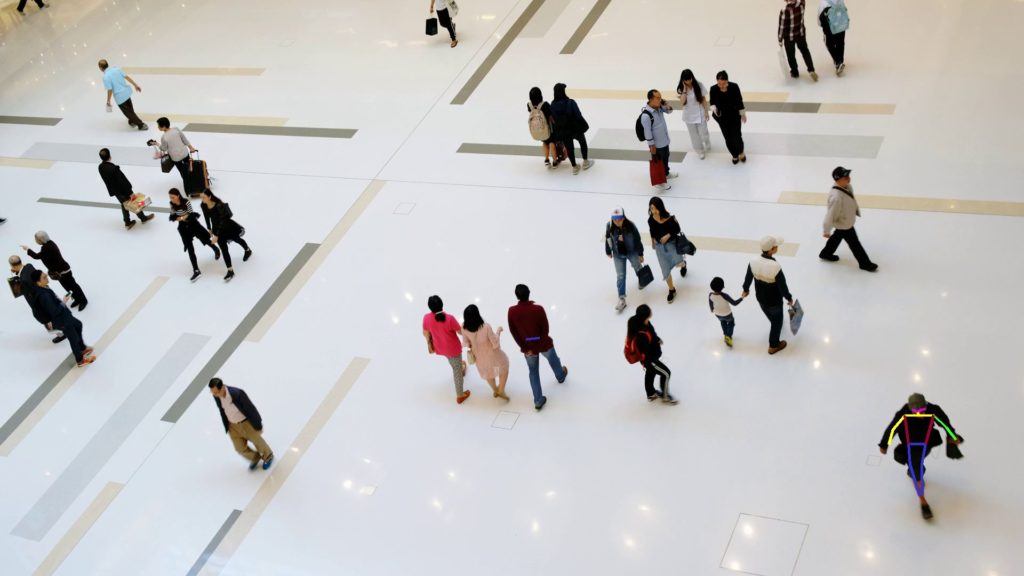
Since we wanted to run inference on real-world CCTV camera images and there was no TensorRT model available that could work properly with CCTV data, we had to create one from scratch. So, we moved to the next approach.
1.2. OpenPifPaf
OpenPifPaf is the official implementation of a paper titled “PifPaf: Composite Fields for Human Pose Estimation” by researchers at the EPFL VITA lab. According to the paper, it “uses a Part Intensity Field (PIF) to localize body parts and a Part Association Field (PAF) to associate body parts with each other to form full human poses.” Here is a sample image of how OpenPifPaf works:
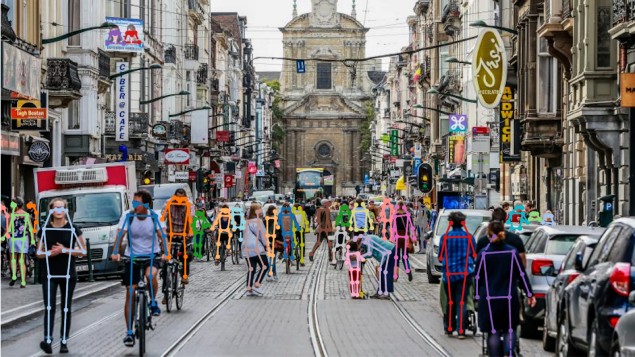
Since OpenPifPaf is optimized for crowded street scenes, it works well on CCTV frames (Figure 1) as well as images captured from a close distance (Figure 6). Therefore, we continued working with OpenPifPaf to run pose estimation on real-world CCTV data.
2. Deploying OpenPifPaf pose estimator on Jetson platforms
Since the official OpenPifPaf repository uses PyTorch Framework, we applied the steps in Figure 5 to optimize the OpenPifPaf model to be able to run it on Jetson devices.

Figure 5. Deploying a PyTorch model On Jetson Device steps
1- Train a PyTorch Model:
For the first step, we used the pre-trained model of OpenPifPaf version 0.12a4 for TensorRT version 7 and OpenPifPaf version 0.10.0 for TensorRT version 6.
2- Export an ONNX model from the PyTorch model:
We have used ONNX (stands for Open Neural Network Exchange) as an intermediate (go-between) format to convert different deep learning models that are trained on various frameworks. We have utilized the `export_onnx` module of OpenPifPaf to export an ONNX model from the OpenPifPaf Pytorch model for this step. We had got some errors by running that module on Jetson Platforms, so we exported two ONNX models with different input sizes on an X86 device from OpenPifPaf version 0.12a4 and uploaded them on Galliot-Models to be available for download from Jetson devices.
3- Generate a TensorRT Engine from the ONNX model:
It is required to generate a TensorRT Engine in the third step for executing high-performance inference on Jetson devices. TensorRT achieved the optimization by converting the model to FP16 (16-bit floating point) or INT8 (8-bit integer) instead of the usual FP32. However, it causes a slight decrease in accuracy; it still yields significant improvements to inference time speed, performing some hardware-specific transformations and modifying model architecture to speed up the inference time. We used the ONNX parser from ONNX-TensorRT project tag 7.0 to build a TensorRT engine with Jetpack 4.4 (TensorRT 7.1.3).
4- Run inference from the TensorRT Engine:
After building the TensorRT inference engine, we prepared the inference code. The inference code consists of a pre-processing module, an inference module, and a post-processing module. We pre-processed the data by applying normalization, ran inference using this inference module, and customized the OpenPifPaf post-processing module to decode the model output.
The inference module consists of the following steps:
1- Allocate buffers for inputs and outputs in the GPU.
2- Copy data from the host to the allocated input buffers in the GPU.
3- Run inference in the GPU.
4- Copy results from the GPU to the host.
5- Reshape the results
We have gathered all the required packages, frameworks, and codes of the above-mentioned steps in a Dockerfile to be easily used to run our optimized OpenPifPaf-TensorRT Pose-Estimation on a Jetson board that has Jetpack 4.4 installed.
Technical Note: Input Size Matters
We exported two ONNX models with different input sizes from OpenPifPaf version 0.12a4, the smaller one with (321,193) input size and the larger one with (641, 369) input size.
The network with the smaller input size worked well on images containing larger objects but failed to generalize to CCTV-like data. This model consumed less inference time and was faster than the other model.
However, the other network worked great on images containing smaller objects, like CCTV images with small objects. It also did well on images with larger objects, but this model was slower than the previous model.
We continued working with both models to implement each one for its specific use case.
We have tested our OpenPifPaf-TensorRT application on a surveillance video on RTX 2070, Jetson TX2, and Jetson Nano which you can see the results in Table 1.
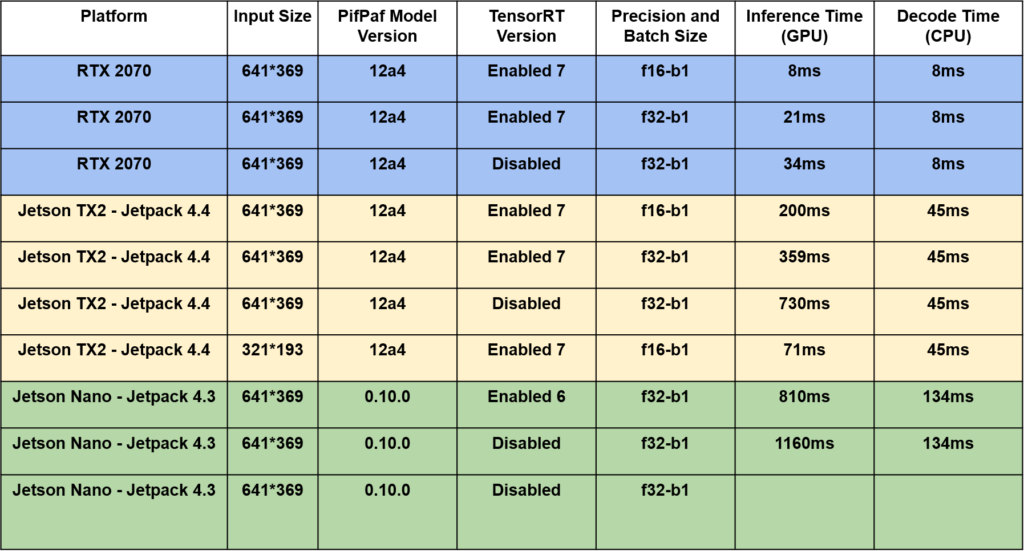
As you can see, this model works great with images captured from a close distance to the subject as well as real-time CCTV images.
This is the first time that the OpenPifPaf, which is a complex and heavy model, has been deployed on Jetson devices.
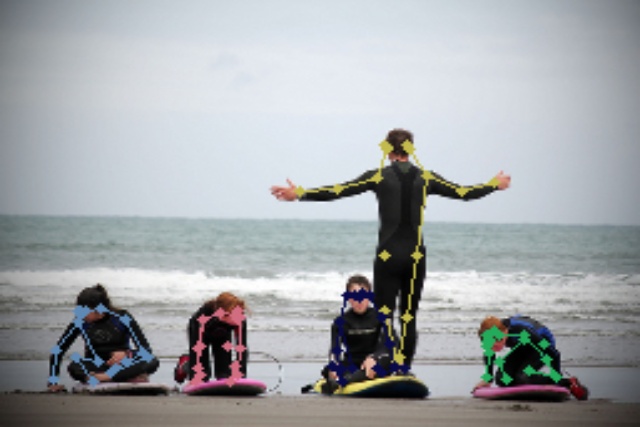
3. Conclusion
Deploying complex deep-learning models on edge devices with limited memory is challenging. In this post, we have explained how we couldn’t find a well-trained pose estimation model on CCTV cameras for Jetson devices and described the challenges we have faced in going through the process and solving the problem. Accordingly, we came up with a solution that, to the best of our knowledge, is the only pose estimation model deployed on Jetson that works well on CCTV images.
Moreover, we tried to walk through the steps to run pose estimation on Jetson platforms by building a TensorRT inference engine from an OpenPifPaf PyTorch model. In future works, we aim to optimize the pose estimation model to achieve higher inference speed to run pose estimation on input videos in real-time.
It is worth mentioning that we have also used this developed model deployed on Jetson for Galliot’s “Smart Social Distancing” and “Face Mask Detection” products.
If you have deployed our OpenPifPaf-TensorRT model on any of your applications, we encourage you to share your thoughts and experiences with us in the comment section at the end of this article. You can also get in touch with us through “hello [at] galliot [dot] us” for further questions.
Leave us a comment
Comments
Get Started
Have a question? Send us a message and we will respond as soon as possible.
Do you guys have a docker image for it? I would love to test it on my TX2 but building every library from source is a nightmare and every development that I try out requires different builds.
Thank you for your comment, Marcus. You can refer to our GitHub repo for instructions on how to build the Docker file for Jetson TX2 with minimal effort. You’ll need Jetpack 4.3 to build this Docker file. We will also push the Docker image to Docker Hub soon.
This is a excellent website, might you be interested in doing an interview regarding how you developed it? If so e-mail me!
Hi Greg, Please reach out to us at hello@galliot.us
Links for the OpenPifPaf deployment isn’t working, can you guys please help here:)
Hi Shubham. The related codes and materials have been moved to this repository: https://github.com/galliot-us/PifPaf-TensorRT-Pose-Estimation
Excellent work, Thank you for this article, but I have a problem, I’m trying to install the docker on Jetson Nano with JetPak 4.4 following the instruction on README but I get errors during onnx-tensorrt installation: /onnx-tensorrt/NvOnnxParser.h:66:8: error: ‘int32_t’ does not name a type; did you mean ‘__int128_t’? and others errors that make compliation failed. I’m missing something? Can you help me?
i exported shufflenet onnx to trt, but have errors at pointwiseV2Helpers.h, with invalid resource handle…
I also found that the exported onnx file from openpifpaf is much smaller than the neuralet zoo…. any hints?
Hi, I would like to try this TensorRT model using deepstream SDK. I am unable to get inference output after creating pipeline. Do you guys have any deepstream implementation for pose estimation (python would be preferable)